Psychological Insights From Text Data For Digital Health
Evaluate stress, anxiety, cognitive load, and therapeutic alliance directly from text, without patient questionnaires.
Our models utilize LIWC, the world's most extensively validated language psychology framework, developed by Receptiviti co-founder Dr. James W. Pennebaker.
LIWC has been validated by panels of psychologists, and is cited in over 20,000 studies, many of which focus on mental health, coping, recovery, and therapy outcomes.
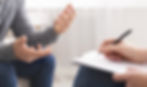
How is Receptiviti used by healthcare providers?
Measurement-based care​
Collecting data is a key step toward better understanding patients, forecasting likely trajectories, and ensuring the greatest likelihood of positive outcomes. Receptiviti converts unstructured language data into 100+ datapoints that reflect a person's cognitive state, temporal and attentional focus, emotions and more.
​​
​
Measure the therapeutic alliance​
According to Lambert and Barley (2001), 30% of patient outcomes in psychotherapy can be attributed to the therapeutic alliance and facilitative conditions, such as empathy, warmth, and congruence. Receptiviti measures empathy by evaluating language and psychological synchrony between healthcare providers and patients. Learn more
​​​​​
​
Improve adherence by customizing communications patients' cognitive styles
By analyzing patient language, Receptiviti uncovers how individuals make decisions and interpret information, and makes it possible for providers to tailor communication with each patient to increase engagement and improve adherence.
Extensively validated and cited in thousands of mental health and therapy-related publications
Receptiviti's models have been constructed and extensively validated by panels of psychologists and have been cited in over 19,000 research studies -- many of which focus on understanding the linguistic fingerprints of mental health, coping, disease, recovery, and treatment delivery. Here's a small sample of the research:
Why is Receptiviti uniquely suited for digital health use cases?
​
Because traditional NLP techniques discard all the words that contain the most important indicators for understanding psychology, social processes, and mental health.
​
Experts in psychology and linguistics have long recognized how significant and predictive people’s use of function words can be. In thousands of peer-reviewed studies over the past 20 years, researchers have proven the predictive value of function words, and the important role they play in understanding psychology, personality, wellness, emotions, interpersonal and group dynamics.
Receptiviti incorporates stop word analysis (SWA) and identifies language-based psychological indicators associated with mental health and distress-related concerns such as depression, stress, and social anxiety, and also enables measurement of the therapeutic alliance - one of the most important predictors of treatment success.
Our models have been constructed and extensively validated by panels of psychologists and have been cited in over 19,000 peer-reviewed research studies, many of which focus on understanding the linguistic fingerprints of mental health, coping, disease, recovery, and treatment delivery. And, in a recent study conducted by researchers at Leiden and Utrecht Universities, Receptiviti outperformed BERT, 95.2% vs. 60.7%, in predicting mental disorders from language.