Receptiviti has developed a prototype tool to showcase to marketing and PR-focused organizations. The tool combines analysis of language data via our API's scientifically validated psycholinguistic dimensions with very targeted Large Language Model (LLM) prompts to produce actionable insights. These insights empower marketing and PR professionals to develop content that resonates effectively with target consumers, going beyond demographic variables and traditional brand voice metrics by basing marketing and communication strategies on psychological factors that influence consumer behavior.
Below we provide descriptions and screenshots of the tool as a showcase. For a live demo, contact us.
Receptiviti and Marketing

PR and marketing organizations and platforms use Receptiviti to analyze audience and brand-generated content to produce psychographic insights. This analysis characterizes market segments and evaluates brand voice, enabling marketing professionals to enhance ad personalization and marketing strategies with a data-driven approach.
Why Do LLMs Fall Short for Marketers?
LLMs excel at generating descriptive language, summaries, and interpretations, but they are ill-suited for analyzing personality and psychology. LLMs rely on patterns in training data rather than established psychological frameworks. This limits their ability to produce psychographic insights about brands and audiences that are credible, repeatable, and rooted in scientific rigor.
Psycholinguistics and LLMs for Automated Marketing Insights

Receptiviti’s psycholinguistic dimensions provide the foundation for making LLMs effective in producing actionable marketing insights. Our scientifically validated dimensions ensure consistent, reliable measurement of psychology from language. By combining Receptiviti’s scores with LLMs, marketers can automate audience
segmentation and brand voice analysis, unlocking the full potential of AI-driven marketing strategies while maintaining accuracy and transparency of insights.
Part 1- Running the Audience Segmentation Analysis
The Example Dataset
The example dataset consists of publicly-accessible social media posts written by individuals who are grouped into broad consumer segments based on the communities they commonly post in. Here, we focus on language data written by individuals who, based on their post history, are likely sports and outdoor goods shoppers.
Scoring Language Data with the Receptiviti API
The tool scores the dataset via the Receptiviti API, evaluating each individual's psychological profile based on Receptiviti’s Needs & Values and Big Five personality dimensions.
Cluster Analysis
The tool uses a clustering algorithm on Receptiviti scores to identify three statistically significant psychologically distinct market segments, assigning each individual to a market segment based on shared traits. By averaging the scores for individuals, the tool identifies key characteristics of each market segment.
Score Translation
The average scores for each market segment are translated into categories, such as "highly extraverted" or "moderately open" to make the results interpretable by LLMs, which cannot process raw numerical data (i.e., scores).
LLM Prompts
The translated scores, along with details about the consumer segment, product, and Receptiviti measures, are incorporated into structured prompts for the LLM. These prompts not only capture psychological insights but also provide expert guidance on how traits influence communication preferences and behavior. This enables the LLM to generate market segment profiles and tailored communication recommendations based on Receptiviti data.
Part 2 - Audience Segmentation Results
The cluster results based on Receptiviti scores are illustrated in a scatter plot, with bar charts highlighting differences between the market segments. The LLM effectively summarizes the Receptiviti results, outlines the unique attributes of each market segment, and provides communication recommendations. These insights offer marketing and PR professionals a nuanced understanding of their target consumers, including insights into their identity and characteristics and the underlying factors driving their decision-making.

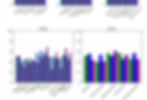

Part 3 - Running the Brand Content Tailoring Analysis
The Example Datasets
The Target Brand Voice: We collected public brand-generated content from North Face’s website. This text is analyzed using the Receptiviti API to identify the brand’s projected personality, tone, and communication style.
The Target Audience Segment: We select a market segment from the list of segments that was generated using the audience segmentation process described earlier.
Generic Ad Copy: We upload generic ad copy for a sports and outdoors product. This text is also analyzed by the Receptiviti API to determine the brand voice associated with the copy.
LLM Prompts
The LLM is prompted to produce copy for “luxury glamping accessories” by transforming the original generic ad copy’s language to better align with the target brand voice (i.e., North Face) and implementing communication style recommendations that are likely to help the copy better resonate with the selected segment of consumers (i.e., The Blue Audience Group).

Part 4 - Brand Content Tailoring Results
The LLM transforms the generic ad copy (i.e., input text) to reflect a tone that embodies a greater degree of openness to change, friendliness (i.e., a subfacet of extraversion), and an emphasis on new experiences - traits Receptiviti identified as important to the Blue Group. The bar chart compares the Receptiviti scores of the original ad copy and the revised version, offering a clear evaluation of how effectively the LLM aligned the content with the target brand voice and audience segment.
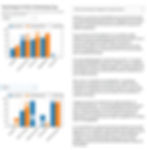
Receptiviti’s metrics provide a critical layer of transparency and accountability, enabling marketers to assess the LLM's revisions. Additionally, these scores can serve as a feedback loop, guiding the LLM to further refine the ad copy by adjusting specific elements that Receptiviti identifies as misaligned.
What Else Is Possible with Receptiviti and LLMs?
This demo is just one example of what is possible by combining Receptiviti’s powerful psycholinguistic dimensions with the generative capabilities of LLMs.
Since Receptiviti can analyze language data from virtually any source, there is potential to explore other types of audience and brand-generated content beyond social media posts and website copy. LLM prompts can also be tailored to produce insights most relevant to your goals. For instance, instead of focusing solely on differences between audience clusters, you might prioritize identifying similarities to craft a content strategy that resonates with the entire consumer group. Alternatively, you could shift the focus to brand voice evaluation, generating insights to assess whether the brand’s projected personality aligns with its target audience. Or, if you're interested in determining other aspects of consumer and marketing psychology, such as decision-making styles or motivations, this can be explored further through other Receptiviti psycholinguistic frameworks, including our Fast and Slow Thinking (i.e., System 1 and 2 Thinking) and Needs and Values frameworks.
How Can You Get Started?
By combining Receptiviti's validated dimensions and LLMs' generative text capabilities, you can create tools that automate the production of meaningful and scientific marketing insights.
Replicate our prototype by integrating the Receptiviti API with the LLM of your choice to generate insights tailored to your needs and use cases. Whether you are aiming to optimize content creation, improve ad targeting, or streamline analysis, our API serves as a powerful foundation for innovation.
Contact us to learn more about how our API can elevate your projects and transform your approach to marketing and communication analysis.