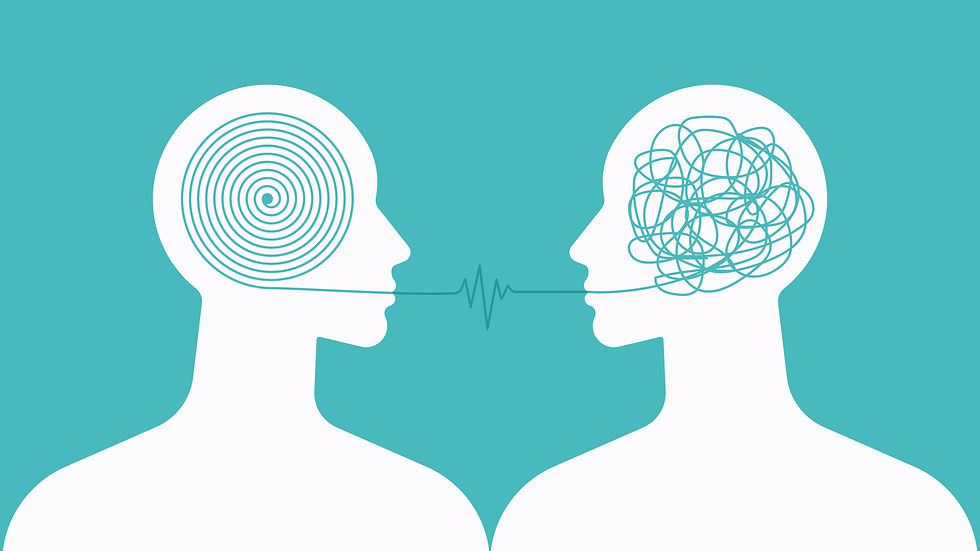
Effective therapy is, in large part, dependent on the therapeutic alliance or bond between patient and therapist. Research demonstrates that a therapist’s ability to exhibit empathy with their patient predicts positive treatment outcomes.
For digital mental health platforms, the language used by the trained therapist, coach, or chatbot during online communication with a patient should embody qualities, much like empathy, that promote the therapeutic alliance and successful treatment.
In fact, research shows that therapists using a similar language style to that of their patients’ more strongly predicts empathy than even traditional reflection. A growing body of research demonstrates how greater language style matching between patients and therapists serves as a reliable and valid indicator of not only the therapeutic alliance but of mental health outcomes as well. In this how-to article, we will explain language style matching, how to calculate it, and its potential applications for digital mental health.
What is language style matching and how can you calculate it?
Language style matching (LSM) is a measure of function word similarity between two people or two texts. In calculating LSM, one must first determine the frequencies of nine function word categories (articles, prepositions, auxiliary verbs, adverbs, quantifiers, conjunctions, negations, as well as personal and impersonal pronouns) present in, for example, a patient’s and therapist’s language during an online session. These function word frequencies can be obtained using Receptiviti’s LIWC framework.
Once the language frequencies are acquired, a distance equation can be calculated for the nine function word categories. The equation below illustrates how to compute the similarity between a therapist’s and patient’s rate of adverbs during their online session. To calculate the total LSM score between the therapist and patient, one would compute the average LSM score for each of the nine function word categories.

Why measure function words?
Measuring function words is useful when assessing various aspects of human thought, emotion, and behavior because we are not typically aware of our own use (as well as others’ use) of function words in writing and in conversation. Specifically, unconscious language use serves as a better predictor of psychological processes, as it captures natural behavior untarnished by biases, such as social desirability.
How can digital mental health platforms utilize LSM?
Research on LSM—and function word similarity, in general—yields promising results for the digital health industry. In particular, the degree to which language style varies between patients and therapists offers further understanding of the therapeutic alliance and mental health.
Empathy and the therapeutic alliance:
Empathy is often cited as an important characteristic in therapists that aids in further developing the therapeutic alliance. Research demonstrates that empathy encourages a common ground between therapist and patient that can manifest implicitly through linguistic similarity. For example, results indicated that therapy sessions were rated as more empathetic when therapists and patients displayed higher (rather than lower) linguistic similarity.
These findings provide insights on more effective interpersonal dynamics surrounding users and mental health coaches on digital platforms. For instance, mental health chatbots could be trained to use similar function words to that of online users as a way to increase perceived empathy and perhaps, subsequently, help improve user mental health.
Mental health:
In addition to predicting crucial aspects of the therapeutic alliance, LSM between therapists and patients can also inform patient mental health. For example, results of a study showed that greater linguistic similarity between therapists and patients diagnosed with anorexia nervosa correlated with greater patient recovery and lower levels of eating disorder symptoms.
Linguistic similarity is also associated with other psychopathological disorders and, more generally, psychological distress. Of note, research demonstrated that patients diagnosed with depression (who were currently experiencing a depressive episode rather than remission) linguistically matched less with their email correspondents.
The pattern of results illustrated within the aforementioned studies indicate that higher LSM may reflect positive mental health, while lower LSM may reflect negative mental health. However, context may modulate this pattern (e.g., coinciding with negative attentional biases, one diagnosed with depression may linguistically match more with negative rather than positive conversations). With that, digital platforms can incorporate LSM as a supplementary measure to help determine user mental health status.
Social support:
LSM has likewise been implicated as a linguistic marker of effective support. One study examining posts on an online peer-support platform found that users who posted about their negative experiences were more likely to view subsequent supportive peer responses as effective (e.g., helpful in diminishing their stress) if the peer responses matched more with their own language style.
Such findings suggest that common ground established through LSM is important for instilling effective support. Research substantiates that social support acts as a strong buffer against stress and negative mental health. Therefore, digital health providers can utilize LSM to aid in verifying the efficacy of their interventions.
Altogether, LSM can be a useful linguistic metric to help inform digital health. Nevertheless, it is also important to consider that other factors, such as the type of therapeutic treatment or mental health disorder, may impact the degree to which LSM correlates with mental health outcomes or elements of the therapeutic alliance.
You can read more about LSM in our research library - it contains many papers that demonstrate LSM in therapy-related use cases. Or contact us to learn more about LSM or using Receptiviti in healthcare.